Artificial Intelligence vs. Machine Learning: Key Differences Explained
Discover the crucial distinctions between Artificial Intelligence (AI) and Machine Learning (ML). Learn why understanding the difference between AI and ML is essential for navigating today's tech-driven world.
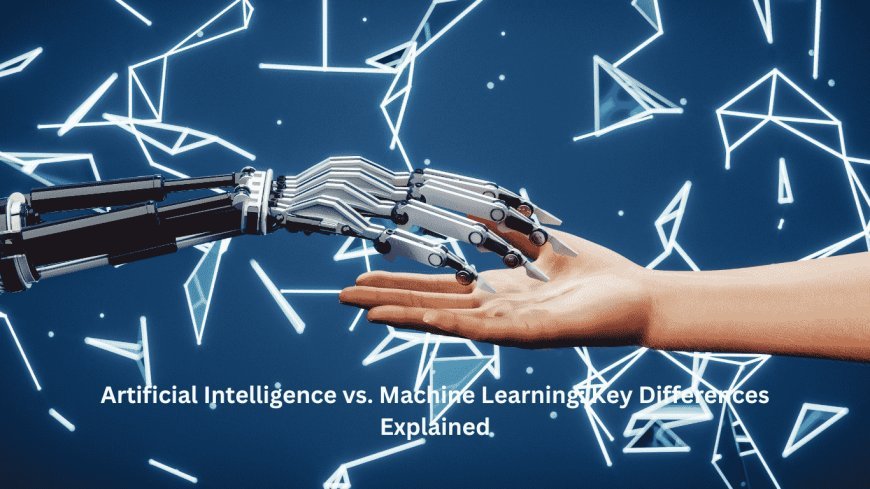
Introduction
Today's tech buzzwords are AI and ML. Though commonly used interchangeably, these phrases refer to separate concepts with different applications and effects. Anyone who wants to keep up with technology must understand AI and ML. Let's discuss AI and ML, why they're important, and how they're changing healthcare and banking.
The Difference Between AI and Machine Learning?
Artificial intellect and Machine Learning both strive to mimic human intellect, but they use distinct methods and serve different purposes. A breakdown:
AI: AI refers to machines that can do tasks in a “smart” fashion. Think of AI as the umbrella term for intelligent systems.
ML: ML is a subset of AI that lets machines learn from data. ML models improve by finding patterns in data rather than obeying rules.
AI is the process of making machines smarter, and ML is one of the key tools.
Artificial Intelligence Definition
Artificial intellect includes many technologies that mimic human intellect. AI can be categorized by its capabilities and scope:
Narrow AI (Weak AI): This AI is designed for picture recognition or language translation. Siri and Alexa are narrow AI. They're good at their jobs but can't do anything else.
General AI (Strong AI): Machines with human-like intelligence in all fields and independent thinking and decision-making are the theory of general AI. Speculative AI like this is regularly shown in sci-fi movies.
Superintelligence: AI surpassing human intelligence and capabilities. Tech ethics and science fiction often discuss superintelligent AI, yet it's far off.
An example of narrow AI in action: Google's Go AI system beats humans.
Understanding ML
AI's Machine Learning subset lets machines learn from data without programming. ML algorithms learn from data sets to predict or decide without being instructed how.
Machine Learning has numerous types:
In supervised learning, algorithms are trained on labeled data with output labels for each training sample. A model can learn to distinguish cats and dogs by being shown labeled photographs.
Unsupervised Learning: ML with unlabeled data. The algorithm searches for data clusters without labels. Unsupervised learning is often used to cluster marketing consumer profiles.
An agent learns by interacting with its environment and obtaining rewards for certain actions in reinforcement learning. In robotics and gaming, systems learn via trial and error to get the best results.
Netflix's recommendation engine employs ML to assess viewing behavior and recommend content.
AI vs. Machine Learning: Key Differences
FeatureAIMachine Learning
ScopeWide range of technology and goalsNarrow—data-driven learning AI
GoalTo mimic human intelligence and fulfill tasksTo learn and predict from data
Applications include robotics and virtual assistants.Applied to predictive analytics, recommendations, etc.
FlexibilityProgrammable AI can handle complicated jobsBig data is needed for ML to progress.
ExamplesSelf-driving automobiles, facial recognitionAnti-spam, recommendation systems
Why Knowing the Difference Matters
Knowing the distinction between AI and ML goes beyond semantics. It affects tech development and corporate strategy. Why it matters:
Business Strategy: Companies must decide whether to use a full AI solution or an ML model for data jobs. This impacts investment, recruiting, and strategy.
AI ethics is a vast field that covers data privacy, algorithmic bias, and employment displacement. These ethical debates are clarified by distinguishing AI and ML.
Resource Allocation: AI systems, especially ML-based ones, require computational power, storage, and data. Knowing what AI technology your project needs optimizes resources.
AI and ML demand different skills. AI developers need a comprehensive computer science background, while ML specialists focus on statistical models, data analysis, and algorithms.
Real-World AI/ML Applications
AI in healthcare may evaluate medical imaging, anticipate disease outbreaks, and aid in surgery with robotic precision.
Based on patient history and data, ML models diagnose diseases and create individualized treatment strategies.
2. Finance AI: Chatbots provide banks customer care and fraud detection systems detect questionable transactions.
Machine Learning algorithms power credit scoring, investment predictions, and tailored financial advise.
3. E-commerce AI: Improves user experience with virtual shopping assistants and customer assistance.
Recommendation engines use ML to make personalized product suggestions based on browsing and purchase history.
4. Manufacturing AI: Predictive maintenance lowers downtime by proactively alerting engineers to possible concerns.
By studying historical data and forecasting demand trends, ML models may optimize supply chain management.
AI/ML Future Trends
Emerging trends in AI and ML could shape the next decade:
AI Democratization: TensorFlow and PyTorch allow small companies and individual developers to build AI apps.
Explainable AI (XAI): Transparency is needed as AI makes more essential decisions. XAI seeks to simplify AI systems in finance and healthcare.
AutoML: Users without coding skills can create ML models using AutoML tools.
Legal frameworks for responsible AI use are being developed by governments and organizations to balance innovation with ethics.
One last thought
AI and ML are changing our lives, work, and relationships. Although they're similar, understanding their variances helps you appreciate their technology functions. As a company leader, developer, or futurist, understanding AI and ML and where they differ can help you make smart decisions and stay ahead in a tech-driven society.
1. Is Machine Learning the same as AI?
Machine learning is a subset of AI that learns from data, while AI encompasses more technologies that replicate human intellect.
2. Can AI exist without ML?
AI systems don't always employ ML. Rule-based AI follows rules rather than learning from data.
3. Why Machine Learning Matters?
ML is essential for data-driven system improvement, making recommendation engines and predictive analytics viable.
4. Examples of AI without ML?
AI without ML includes scripted virtual assistants and rule-based games.