Top 10 Artificial Intelligence and Machine Learning Trends to Watch in 2024
Discover the top AI and machine learning trends shaping 2024, from AI-powered personalization to Edge AI, cybersecurity, autonomous vehicles, and ethical AI. Stay ahead with insights on how these innovations impact industries and daily life.
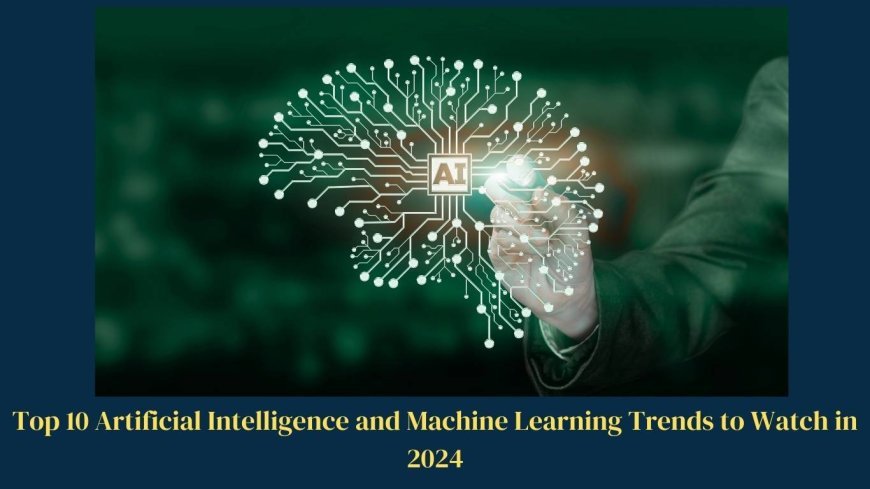
Top AI and Machine Learning Trends to Watch in 2024
Since the launch of ChatGPT in late 2022, the world of artificial intelligence (AI) has seen big changes. In 2023, we moved from testing these technologies to actually using them in real-life applications. Now, as we enter 2024, AI development is getting more mature, with companies paying close attention to safety, fairness, and following new rules. Here’s Top AI and Machine Learning Trends to Watch in 2024-
1. Multimodal AI
Multimodal AI combines multiple types of input—text, graphics, and sound—to provide sophisticated and realistic interactions. This ability to manipulate and process vast amounts of data makes AI more compatible with the integration of human emotions. For example, OpenAI's GPT-4 demonstrates a wide range of capabilities through information-based object visualization or queries through audio input in response to a wide range of AI applications emerging in healthcare, where patient histories and medical image data, and agency planning come together. in between, where access is extended to policy and coding tasks.
2. Agent AI
Agent AI marks a significant shift from systems working reactively, to those that can proactively, and often entirely, work without human input in executing tasks. In contrast to classic AI, which needs guidance in the form of end user input, agentic AI gathers information in an outright manner, recognizes trends, and works accordingly. For instance, agent AI may be used for assessing environmental hazards or overseeing investment portfolios and adapting in real-time. The processes of many systems and tools, along with AI, present an opportunity to transform the way things are developed to provide advanced capabilities that can be invoked with the use of basic syntax.
3. Flexible AI
Open source AI has facilitated global access to powerful models through cost cutting and allowing more interaction within its community. The same year saw the emergence of works like Stable Diffusion and Meta's Llama 2, which precipitated an unprecedented level of collaboration among developers on platforms such as GitHub, therefore allowing even non-advanced AIs Open source AIs have a culture of supporting both accountable refinements and improvements as well as resource lessons for domestic researches, although Dangers associated with fake news and other bad use cases are present.
4. Retrieval-Augmented Generation (RAG)
RAG responds to one of the challenges featured in artificial intelligence: hallucinations, or the generation of false but convincingly looking information by the AI. RAG minimizes model size while improving artificial intelligence answer accuracy through a combination of text generation and information retrieval. Enterprise applications that demand factual accuracy in real time like customer service or reviewing documents greatly benefit from the RAG approach and it bridges the gap to the more enterprise-friendly AI systems.
5. Customized Enterprise Generative AI Models
Enterprises are adopting a smaller range of bespoke AIs that fit business needs rather than large scale general purpose AIs. The given models are all cost effective, less time consuming and protect the data better. For these industries which have developed their own languages such as finance, health care and the legal field, suitable models are built which fulfill the business environment allowing AI to solve its purpose while safeguarding the business from insecurity and regulations.
6. Increased Need for AI and Machine Learning Professionals
As machine learning and other AI features cope with critical enterprise functions, the eager need for skilled AI and machine learning practitioners is still very high, especially for the areas of MLOps, Data Science, and AI programming. These experts have an important role in scaling up and sustaining AI applications in the business. More so, organizations are practicing inclusive design in AI teams to lessen the risks of data bias arising from unequal representation during training; hence a more responsible progression of AI.
7. Shadow AI
Shadow AI continues rising, which means employees using AI inappropriately. There is a tendency for more and more non-technical employees to play around with AI, even when it has not been sanctioned by the IT department; this very well might expose the organization to risks of loss of sensitive information contained in public AIs. It is anticipated that organizations will put in place governance framework in order to contain this trend while encouraging the employees’ creativity where needed while safeguarding once again the data that is required to be safeguarded.
8. Generative AI Reality Check
Businesses have found out the ways of integrating the generative AI into their work, as the first hype begins to fade. Many of the organizations concentrate on recognizing the flaws of AI and making prudent expectations and more importantly building the provisions that will enable the usage of the AI that is within the desired goals of the organization.
9. Increased Focus on AI Ethics and Security
Deepfake technology is now available and very sophisticated cyber attacks are being supported by AI, thus ethical and security issues become paramount. AI detection techniques are still in the infancy stage thus companies must pre-screen AI models for clarity and fairness to reduce risks. Monitoring and guidance are prerequisites for ethical AI deployment to avoid biases or security lapses in its usage.
10. Evolving AI Regulation
The year 2024 marks an important turning point with respect to AI legislation. The provisions of the EU’s AI Act and existing frameworks such as GDPR are creating new benchmarks for compliance expectations, encouraging firms around the world to embed privacy and control over AI systems in their design. In the USA, as exemplified by the executive order on the safe deployment of AI issued by President Biden, it is apparent that the government has endorsed deployment and compliance with regulatory frameworks before AI systems are put into operation. With increasing ai regulation, ethics, security and compliance issues are expected to be standard features in all corporate strategies relating to the deployment of Ai technologies.
Frequently Asked Questions (FAQs)
1. What is multimodal AI?
-
Multimodal AI handles different types of input, like text, images, and sound, which makes it more versatile.
-
Uses include personalized healthcare, customer service, and smart home systems.
2. How is agentic AI different from regular AI?
-
Agentic AI acts on its own to achieve goals without waiting for human input.
-
Applications include environmental monitoring and automated financial management.
3. Why is open-source AI important?
-
Open-source AI makes advanced tools available to more people and encourages ethical development.
-
It allows smaller businesses to use and customize AI tools at a low cost.
4. What is retrieval-augmented generation (RAG), and why is it important?
-
RAG helps AI retrieve information from trusted sources, making responses more accurate.
-
This approach is beneficial for businesses needing reliable, up-to-date information.
In 2024, AI is set to grow even more. With these trends, companies can look forward to smarter, safer, and more ethical AI tools, making technology work better for everyone.